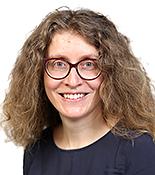
Anna Kreshuk
Group Leader/ Acting Deputy of the Head of CBB.
anna.kreshuk [at] embl.de
ORCID: 0000-0003-1334-6388
EditMachine learning for bioimage analysis
Group Leader/ Acting Deputy of the Head of CBB.
anna.kreshuk [at] embl.de
ORCID: 0000-0003-1334-6388
EditMachine learning is advancing the state of the art in image analysis more rapidly than ever before: for many problems in natural image analysis, automated methods are now approaching parity with humans. One of the major advantages of learning-based approaches is their general applicability: tailoring to a particular problem is performed by providing suitable training data, while the core of the algorithm remains unchanged. Our aim is to build on the latest advances of machine learning and computer vision to develop new methods for the analysis of microscopy images. To bring these methods to members of the life science community without computer vision expertise, we have developed a toolkit for interactive learning and segmentation (ilastik).
While the algorithms in ilastik generalise to provide user-friendly solutions to a wide array of image analysis problems, the most challenging bioimage datasets require a tailored approach. Our group is particularly interested in solving challenging segmentation problems for light or electron microscopy (LM or EM), in 3D and at large scale. Most recently, we have developed methods and tools to segment all cells and nuclei in a juvenile worm of the species Platynereis dumerilii (EM, Vergara et al., Cell 2021), as well as in various plant organs and tissues (LM, Wolny, Cerrone et al., eLife 2020).
All machine learning algorithms require user guidance at the training stage, but deep learning – the driver of the current computer vision revolution – is even more annotation hungry. This problem is especially acute in biological imaging, where annotation of ground-truth data cannot easily be outsourced to non-experts, and changes in experimental conditions can require retraining. Besides the annotation burden, the training process itself depends upon non-trivial expertise in the choice and tuning of hyperparameters. Our group is currently working on methods and training strategies that would reduce the requirements on the amount of training data, some of the early ideas can be seen in https://arxiv.org/abs/2103.14572, https://arxiv.org/abs/2107.02600 and https://www.biorxiv.org/content/10.1101/2021.11.09.467925v1. We are also interested in creative combinations of deep learning and microscopy (Wagner, Beuttenmuelluer et al., bioRxiv 2020) and learning-based analysis of morphology.