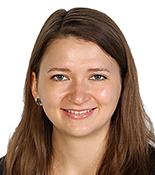
Maria Zimmermann
Group Leader
maria.zimmermann [at] embl.de
ORCID: 0000-0001-6021-1246
EditMulti-omics-based modelling of microbial ecosystems
Group Leader
maria.zimmermann [at] embl.de
ORCID: 0000-0001-6021-1246
EditMicroorganisms live in almost every habitat on Earth and adapt to hostile conditions, such as extreme temperatures, pressures, salinity, pH, or metal concentrations. Microbes are essential for human culture, health, and the environment. In the human body, microorganisms help to digest nutrients and engage in host metabolism, immunity, behaviour, and brain function. With the rise of DNA sequencing technologies, we can now assess the complexity of microbial communities and their genetic potential. For example, the human microbiota comprises hundreds of bacteria, which collectively encode around 100 times more genes than the human genome. However, the functions of more than one-third of these bacterial genes are currently unknown.
Our lab aims to investigate microbial gene functions, especially those relating to metabolism. We combine bioinformatics and mathematical modelling with experimental approaches, such as (meta)genomic and (meta)transcriptomic sequencing, and proteomics and metabolomics (collectively termed multi-omics). We develop multi-omics integration strategies and combine unsupervised clustering, graph-based approaches, and genome-scale metabolic modelling to investigate metabolic adaptations of single microbes and their communities to different conditions. For example, by integrating metabolomics and transcriptomics data from in vitro and in vivo experiments, we identified the core transcriptional response of human gut bacteria to nutrient starvation and mouse colonisation, and discovered regulatory metabolites that help bacteria to cope with stress to increase fitness in the gut (Figure 1).
To quantify microbial community and microbiota–host interactions, we develop mathematical models that describe the underlying molecular exchange processes based on time-resolved and/or spatial metabolomic measurements. Combining in vitro and in vivo experimental data with such models has revealed that gut bacteria can substantially contribute to the host metabolism of medical drugs. With these models at hand, we investigate different scenarios of host–microbiota interactions and predict how perturbations (e.g. nutrients, antibiotics, medical interventions) change microbiota contributions to host metabolism (Figure 2). We are further expanding these approaches to improve our understanding of how dietary and neuroactive compounds affect microbes and their interactions with the host.
The composition and metabolic operation of microbial communities can vary drastically with changes in conditions. By combining computational and experimental strategies to explore metabolism of single microbes and their communities, we aim to identify factors determining microbial community functions, helping us move a step closer to developing targeted metabolic and genetic strategies to control and modify microbial communities for therapeutic, prophylactic, or environmental interventions.
Our team will continue developing and expanding computational and experimental approaches to: