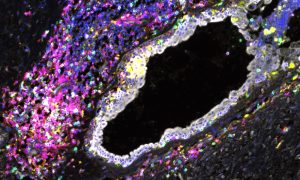
Read the latest Issue
A new statistical method for RNA-seq analysis identifies and corrects for hidden structure between cells, creating order out of seeming chaos.
A new method for analysing RNA sequence data allows researchers to identify new subtypes of cells, creating order out of seeming chaos. Published in Nature Biotechnology, the novel technique developed by scientists at EMBL-EBI represents a major step forward for single-cell genomics.
Single-cell RNA-sequencing is a relatively new technology that helps scientists understand how genes are expressed in different types of healthy tissue and in cancers. It provides data on the gene-expression profiles of hundreds of individual cells in a single experiment, producing an exact picture of the individual cell types. However, the fundamental complexity of single-cell transcriptome profiles has posed a major challenge to making sense of the data.
“With single-cell genomics, we take cells from a tissue and group them into different types based on their expression profile, identifying subtypes that may have a range of functional roles. But to do that properly, we need to deal with confounding factors, and until now we haven’t had robust methods for doing that,” explains John Marioni, Research Group Leader at EMBL-EBI.
The analysis of single cell types is essential for medical research…
A sample from one type of tissue has built-in complexity: some cells will be new and some old, and at any given point in time they will be at different stages of the cell cycle. Most cell types also have hidden sub-types, each of which may have a distinct function. The new single-cell latent variable model (scLVM) allows hidden sub-structure to be detected and controlled for, thereby allowing relevant biological signals to be more easily identified.
“We’ve defined how factors such as cell-cycle stage, measurement noise or biological processes can be taken into account, making it possible to create a more accurate picture of gene expression in different cell types and subtypes,” says Florian Büttner, who led the research at EMBL-EBI as an EMBO Visiting Scientist from the Institute of Computational Biology at Helmholtz Zentrum München. “Combining single-cell analyses with statistical methods lets us identify cell types that would otherwise remain undetected.”
“If all you have is gene expression data from single cells, you need a way to identify and correct for the underlying factors that differentiate individual cells, so you can reveal the underlying biology,” explains Oliver Stegle, Research Group Leader at EMBL-EBI. “Our model accounts for relatedness between single cells, for example whether they are at the same stage of the cell cycle, identifies potentially confounding variables and removes them. It also makes it easier to find new subtypes – variables you might not have known existed – and correct for them, all at one go.”
“The analysis of single cell types is essential for medical research,” asserts Büttner. “Cancer cells, differentiation processes and the pathogenesis of various diseases can be better explored and understood when they are based only on known, detailed cell profiles. Our model now makes it possible to create such profiles using single-cell genomics.”
This post was originally published on EMBL-EBI News.
Looking for past print editions of EMBLetc.? Browse our archive, going back 20 years.
EMBLetc. archive