“Since the launch of the AlphaFold Protein Structure Database, Google DeepMind and EMBL-EBI have engaged closely with the user communities. The feedback we have received highlights a desire amongst many scientists to better understand the capabilities of AI tools like the AlphaFold Database and how they can be most effectively applied in their work. We hope that this course will enable many more scientists to use AlphaFold and the AlphaFold Database to further their research – and ultimately help us build a stronger and more inclusive AI community in the life sciences.” – Sam Miller, Director at Google DeepMind Institute
The AI revolution hinges on accessible training
Sameer Velankar explains why researchers need accessible training to understand and leverage artificial intelligence in the life sciences
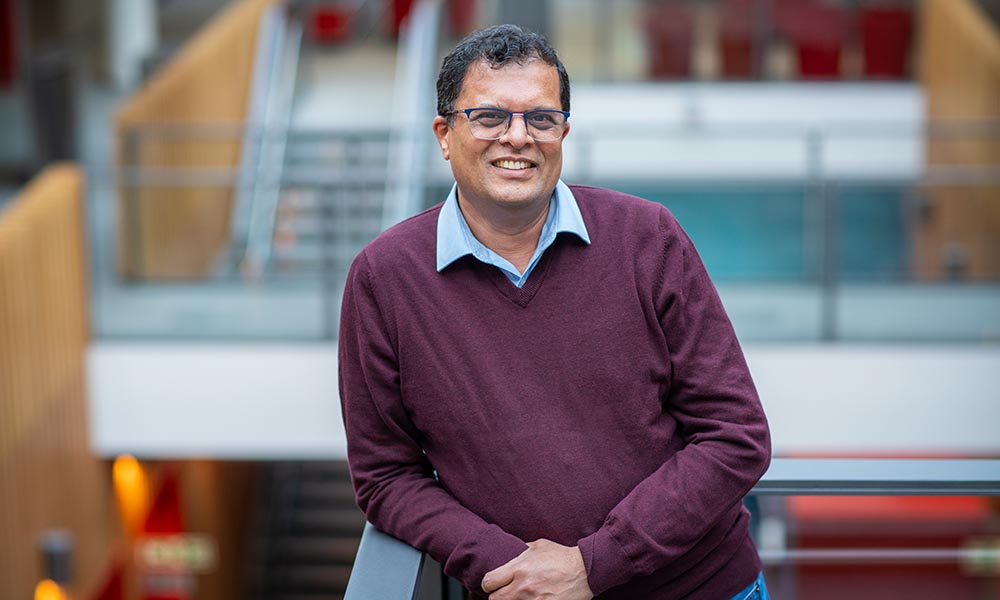
Using any technology to its full potential, whether a basic word processor or a cutting-edge AI algorithm, requires some training. To truly tap into the benefits of technology, users need to understand how it works, grasp its limitations, and employ it responsibly. Nowhere is this more relevant than in the world of AI.
Sameer Velankar, Team Leader at EMBL’s European Bioinformatics Institute (EMBL-EBI), oversees the team that manages the Protein Data Bank in Europe and the AlphaFold Protein Structure Database, two essential resources for structural biology.
Here, Velankar explains how Google DeepMind and EMBL-EBI are actively collaborating to plug the knowledge gaps surrounding the revolutionary AlphaFold AI technology, which has generated structure predictions for almost all known proteins.
Why is it important to provide accessible training for new technologies in the life sciences?
With rapid advances in technologies, accessible training lowers the barriers to entry and enables life scientists around the world to integrate new tech into their work streams effectively and responsibly.
Understanding how to use results from new technologies or databases is not straightforward, and a healthy amount of background knowledge and critical thinking are usually required.
Scientists must assess whether the data they get are useful in a given context. It’s also important for users to be aware of the limitations of technology – what it can and can’t do, what it’s good at, and where it falls short. This is only possible through robust documentation and accessible training.
How would you describe training that is accessible?
Accessibility is multifaceted. At its minimum, training should be easily findable and not behind a paywall. EMBL-EBI has a long history of providing freely available training in an electronic format so it can be accessed by a global audience at no cost.
Accessible training also has to be comprehensive and easy to understand by different users with a variety of training backgrounds, levels of expertise and abilities. This is a continuous process. The only way to navigate this challenge is to continually engage with the community, taking into account feedback and questions from a broad range of users when developing training material and tutorials.
Why do AlphaFold users need training materials now?
Until a few years ago, the availability of protein structure data was limited to a few hundred thousand experimentally determined protein structures, so not everyone had access to a structure model of interest. This meant that not everyone needed to learn how to use structure models effectively. But since Google DeepMind and EMBL-EBI made millions of AlphaFold protein structure predictions publicly available, we have entered a world where structural data is abundant.
This means anyone who needs a 3D structure model for their protein of interest can have one, regardless of whether they are studying human health, crops, biodiversity, enzymes, or something else entirely. And while AI predictions don’t replace experimental data and come in various levels of accuracy, they are a useful tool which the scientific community has been using heavily and creatively. There are already 18,000 scientific papers citing AlphaFold, and the database has over 1.7 million users in 190 countries. More details about AlphaFold’s impact are available in a recently published preprint.
Excitingly, it’s not just structural biologists but also molecular biologists, clinicians, data scientists, and others who are using protein structure models to accelerate their research. AlphaFold predictions are reaching millions of users who have never had much contact with protein structure data before.
So we urgently need to fill the gap in the AlphaFold training material to support scientists wanting to make use of this rich dataset. Google DeepMind and EMBL-EBI are hoping to bridge the gap in training material with the new comprehensive, self-paced, online course they co-developed titled ‘AlphaFold: a practical guide’.
What makes the ‘AlphaFold: a practical guide’ course unique?
For the first time, Google DeepMind and EMBL-EBI have together launched a comprehensive training module with input from experts in different areas of the life sciences. It contains answers to frequently asked questions that users might have about the AlphaFold software and database but were too afraid to ask.
The ‘AlphaFold: a practical guide’ course outlines AlphaFold’s strengths and limitations, different ways of accessing the predictions – including through the AlphaFold Database, examples of how others are using AlphaFold predictions, and its real-world impact so far. We hope this will guide and inspire users to integrate AlphaFold predictions into their workflows in effective ways.
Because the course is modular, it’s easy for learners to focus solely on their areas of interest. The videos, tutorials, and slides featured in the course support different ways of learning.
Importantly, following community feedback, we’ve made great efforts to make the course comprehensible for undergraduate students and upwards.
What are some of the common misconceptions about AlphaFold?
In my experience, there has been some confusion about what AlphaFold can and can’t do. So in this course, we have tried to explain the limitations of the method and whether the predictions are the right things to use in a given context.
For example, we have addressed some of the common questions about the absence of ligands and multimers in the AlphaFold database. A whole section of the course is dedicated to explaining the AlphaFold quality metrics in more detail, specifically the per-residue model confidence score (pLDDT) and the predicted aligned error (PAE), and how to use these to assess AlphaFold models.
What do you hope will be the impact of the new AlphaFold training course?
I hope it will help researchers benefit from AlphaFold predictions in a way that is productive for them and accelerate life science research through well-designed experiments that shed light on biological processes at the molecular level.
We’ve already seen AlphaFold have a real-world impact in a number of disciplines, not only accelerating structural biology and basic science, but also empowering translational research such as understanding proteins linked to disease, vaccine development, and addressing global challenges such as cleaning plastics pollution by creating plastic-eating enzymes. There is a lot more to be uncovered by using this transformative technology in an optimal and responsible way, and this course aims to support and enable that.
Our hope is that this training course can also be integrated into university curricula and that we can continue to improve it and develop it based on community feedback.
“Our goal is to help make AlphaFold more accessible and useful to a wider spectrum of end users. To that end, the course content was designed to be helpful for scientists at all career stages, and with relevance to a broad array of research fields. We look forward to collaborating with EMBL-EBI to further increase global access to AlphaFold and science education.” – Sam Miller, Director at Google DeepMind Institute
What’s next for the team on this front?
Structural biology as a discipline is opening up to experts from other fields. Together with Google DeepMind, we’re planning to further develop the training – covering potential topics such as how to analyse and use experimentally produced and AI-predicted protein structures, as well as the pros and cons of different structure determination techniques.
By bringing all these together in one place, we can create a comprehensive training resource that enables the global scientific community to use protein structures and predictions on the same scale we use genomes or protein sequences. This has the potential to lower entry barriers, increase diversity and collaboration in the field, and support the development of solutions for global challenges.
Explore AlphaFold: a practical guide
‘AlphaFold: a practical guide’ is a new comprehensive, freely available training course co-developed by Google DeepMind and EMBL-EBI. It was developed using feedback from the life science community and is designed to answer any questions you may have about AlphaFold. This includes how to find and use protein structures of your interest, how to interpret their accuracy scores, the limitations of the predictions, and how other researchers are using AlphaFold.