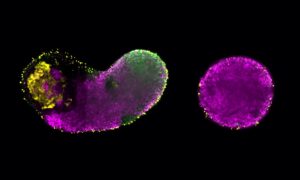
Analysis of human genomes in the cloud
EMBL scientists present tool for large-scale analysis of genomic data with cloud computing.
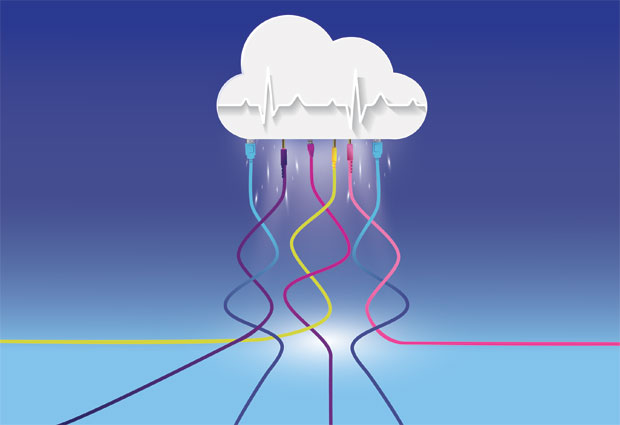
Scientists from EMBL present a tool for large-scale analysis of genomic data with cloud computing. Main advantages of the new tool, called Butler, are continuous system monitoring and its ability to self-heal in case of failure, allowing for 43% more efficient data processing than previous approaches. The tool was developed for the Pan-Cancer project. The team published the method in Nature Biotechnology.
Most bioinformatics software used for genomic analysis is experimental in nature and has a relatively high failure rate. In addition, cloud infrastructure itself, when run at scale, is prone to system crashes. These setbacks mean that big biomedical data analysis can take a long time and incur huge costs. To solve these problems, Sergei Yakneen, Jan Korbel, and colleagues at EMBL developed a system that identifies and fixes crashes efficiently.
Researchers performing analysis on the cloud need a number of technological skills, from configuring large clusters of machines and loading them with software, to handling networking, data security, and efficiently recovering from crashes. Butler helps researchers master these new domains by serving up appropriate tools that overcome all these challenges.
Saving time by checking the system’s pulse
Butler differs from other bioinformatics workflow systems because it constantly collects health metrics from all system components, for example the Central Processing Unit (CPU), memory, or disk space. Its self-healing modules use these health metrics to figure out when something has gone wrong, and can take automated action to restart failed services or machines.
When this automated action does not work, a human operator is notified by email or Slack to solve the problem. Previously, a crew of trained people was necessary to check a similar system and detect failures. By automating this process, Butler dramatically reduces the time needed to execute large projects. “It is indeed very rewarding that these large-scale analyses can now take place in a few months instead of years,” Korbel says.
Open source
Good solutions are already available for individual challenges associated with scientific computing in the cloud. So instead of reinventing the wheel, the team improved existing technologies. “We built Butler by integrating a large number of established open source projects”, says Sergei Yakneen, the paper’s first author, currently Chief Operating Officer at SOPHiA GENETICS. “This dramatically improves the ease and cost-effectiveness with which the software can be maintained, and regularly brings new features into the Butler ecosystem without the need for major development efforts.”
Besides system stability and maintainability, using the cloud for genomics research is also challenging with respect to data privacy and the way it is regulated in different countries. Bigger projects will need to make simultaneous use of several cloud environments in different institutes and countries in order to meet the diverse data handling requirements of various jurisdictions. Butler addresses this challenge by being able to run on a wide variety of cloud computing platforms, including most major commercial and academic clouds. This allows researchers access to the widest variety of datasets while meeting stringent data protection requirements.
Butler in use
Butler’s ability to facilitate such complex analyses was demonstrated in the context of the Pan-Cancer Analysis of the Whole Genome study. Butler processed a 725 terabyte cancer genome dataset in a time-efficient and uniform manner, on 1500 CPU cores, 5.5 terabytes of RAM, and approximately one petabyte of storage. The European Bioinformatics Institute (EMBL-EBI) played a crucial role by providing access and support to their Embassy Cloud, which was used for testing Butler. The system has recently been used in other projects as well, for example in the European Open Science Cloud pilot project (EOSC).
The Pan-Cancer project
The Pan-Cancer Analysis of Whole Genomes project is a collaboration involving more than 1300 scientists and clinicians from 37 countries. It involved analysis of more than 2600 genomes of 38 different tumour types, creating a huge resource of primary cancer genomes. This was the starting point for 16 working groups to study multiple aspects of cancer development, causation, progression, and classification.
Related links
- Pan-Cancer landing page
- Frequently Asked Questions on the Pan-Cancer project
- Korbel group
- SOPHiA GENETICS
- The EMBL-EBI Embassy Cloud
- Research highlight: Scientists identify new genetic drivers of cancer
- Research highlight: Protecting data in the cloud
- Research highlight: Studying DNA rearrangement to understand cancer
- Research highlight: Finding genetic cancer risks
- Research highlight: Rapid and safe analysis of thousands of human genomes in the cloud