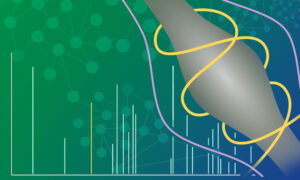
Read the latest Issue
Thousands of new protein structures, predicted using machine learning, are now available in EMBL-EBI's Pfam database
The field of protein structure prediction has greatly advanced in recent years thanks to increasingly accurate deep-learning methods. A new such method, called trRosetta developed by the University of Washington, has now made thousands of protein structures available via EMBL-EBI’s Pfam data resource.
More than 6300 protein structures have been predicted in this way and are now available in Pfam, with more to follow.
“This is a big step forward because it gives the research community open access to thousands of new protein structures predicted using accurate computational models,” explains Alex Bateman, Senior Team Leader at EMBL-EBI. “This new dataset will enable researchers to explore proteins for which the structures remained hidden until now. And by exploring these protein structures, they can also start to gradually understand the protein functions.”
trRosetta is an algorithm for fast and accurate protein structure prediction. It uses the large, multiple sequence alignments available in Pfam and applies a deep learning model to predict the transformations and structure parameters for each protein. It then applies the Rosetta pipeline to predict the structure.
“We are delighted to work with the Pfam team to make our structure models widely available to the scientific community,” says David Baker, Director of the Institute for Protein Design at the University of Washington.
Pfam uses a quality score called the Local Distance Difference Test (lDDT). An lDDT score of 0.6 or greater is considered a reasonable model and scores above 0.8 are great models. The large majority of structural models obtained from rtRosetta are of good quality, with an lDDT score of over 0.7.
The Pfam database provides a complete and accurate classification of protein families and domains. Pfam is used by experimental biologists researching specific proteins, by structural biologists to identify new targets for structure determination, by computational biologists to organise sequences and by evolutionary biologists tracing the origins of proteins.
“It’s great to see so much progress in this field,” says Bateman. “Just 10 years ago, this kind of dataset was something we could only dream of, so to see it become a reality is amazing, and we hope many researchers will explore it and use it in their work.”
This post was originally published on EMBL-EBI News.
Looking for past print editions of EMBLetc.? Browse our archive, going back 20 years.
EMBLetc. archive