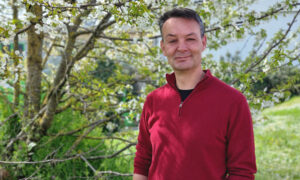
Welcome: Anna Kreshuk
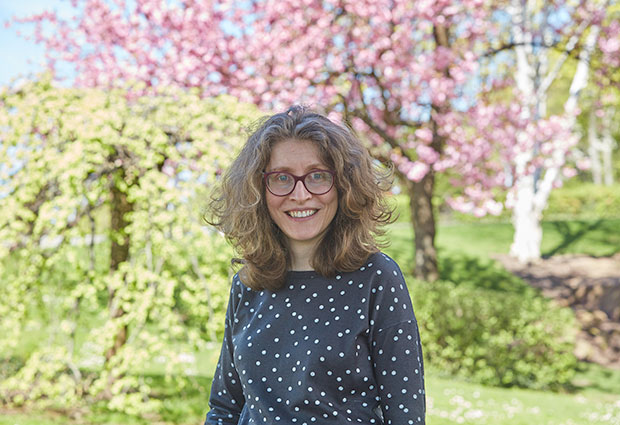
Anna Kreshuk will join the Cell Biology and Biophysics Unit in July, and is already looking forward to collaborations. Her group will use machine learning to develop automated methods to help biologists speed up image analysis.
You studied mathematics, what brings you to EMBL?
The life sciences are so cool! Learning about life, why things happen and how they are built, about what’s really here. Mathematics is aesthetically beautiful and I find it really interesting, but it’s not applied like biology. After my undergrad, I felt maths was a bit too theoretical for me and I wanted to do something of more immediate use. I worked as a computer scientist for some time at CERN and realised I wanted to do research. I decided to do a PhD in computer science, focusing on machine-learning methods that could be applied to the life sciences.
What will you work on at EMBL?
Imaging is extremely important in the life sciences, but right now it’s also a bottleneck. In my group, we will develop machine learning-based methods and tools for automatic segmentation, classification and analysis of biological images. For instance, electron microscopy provides tons of structural information, but depending on the quality of the samples it can take months to analyse the data. Just imagine you had a tool that could extract all that information automatically!
I’m also thinking about other kinds of imaging techniques that can be combined. I want my methods to help biologists see the correlations between different techniques, and to use the information in their follow-up analysis. In general, it’s all about extracting information from images automatically and not having people sit there and do it manually. It’s quite abstract, but it can be used for so many things!
Young scientists need to train themselves to think like a group leader if their ambition is to become one.
What impact would you like your research to have?
I develop methods, so my work is collaborative by nature. I hope my team will be able to automate the most time-consuming parts of biological research and I’d love to see my methods employed to move biology forward. I want to help biologists do things they’re not even considering at the moment because it takes too long to do. By removing the imaging analysis bottlenecks, we’ll enable researchers to think of more interesting and ambitious experiments. I don’t think I could be a wet lab scientist and do the experiments myself, but this is the way I can contribute. I know I won’t get a Nobel prize, but I hope my work will be an important contribution to the work of others – and might be mentioned in someone’s acceptance speech eventually!
What’s the best advice you’ve received in your career?
As a PhD student, there were times when I couldn’t see the road ahead. When your research is not going great, you just have to put one foot in front of the other and keep on going: the view will open when you turn the corner. If something doesn’t work, there will be other things. If you don’t see things right away, it doesn’t mean that you’ll never see them, the road will open up – that’s why it’s important to keep at it.
What advice do you have for young scientists who want to become group leaders?
Becoming a group leader is a very exciting opportunity. When you work in research for a long enough time, you start having more ideas than you can work on, so it’s great to be able to delegate to somebody else to execute them. But if you plan to develop your own ideas you need to see the big picture. I’ve seen many junior scientists working on a project for years. They were able to write the methods section, but couldn’t write the introduction or the related work because they didn’t understand the big picture. Scientists should start working on becoming a group leader long before applying for group leader positions. You need to ask yourself: Why are we doing this? What will be the next step if this works? Or if it doesn’t? How will this push science forward? If we could put three more people on this, what would they do – would it help? Asking these kinds of questions is what you’ll be doing as a group leader. Young scientists need to train themselves to think like a group leader if their ambition is to become one.
What is Machine Learning?
Babies start learning the moment they are born. Whether it is holding a spoon or mastering irregular French verbs, we learn by getting new information and we improve through repetition. But the ability to learn and improve at a task is not exclusively human: computers can do it too.
Machine Learning brings together statistics and computer science so that computers learn to perform a specific task without being programmed to do so. For a computer to learn, it needs to have some initial data on how to do a specific task. The computer will find statistical patterns in the data that will enable it to establish the algorithm by which future data will be sorted. Just like babies, computers use experience to improve at a task. The more (useful) data the machine has access to over time, the more finely tuned its algorithm will become and more accurate decisions it will be able to make. Machine Learning’s ultimate goal is for the algorithm to be able to generalize beyond the training samples and successfully interpret data that it has never ‘seen’ before.
Machine Learning is already widely applied: from email spam filters, autocorrecting your texting mistakes, to suggesting what movie to watch next, you probably use Machine Learning dozens of times a day without knowing it.